Beyond Fairness: Reparative Algorithms to Address Historical Injustices of Housing Discrimination in the US
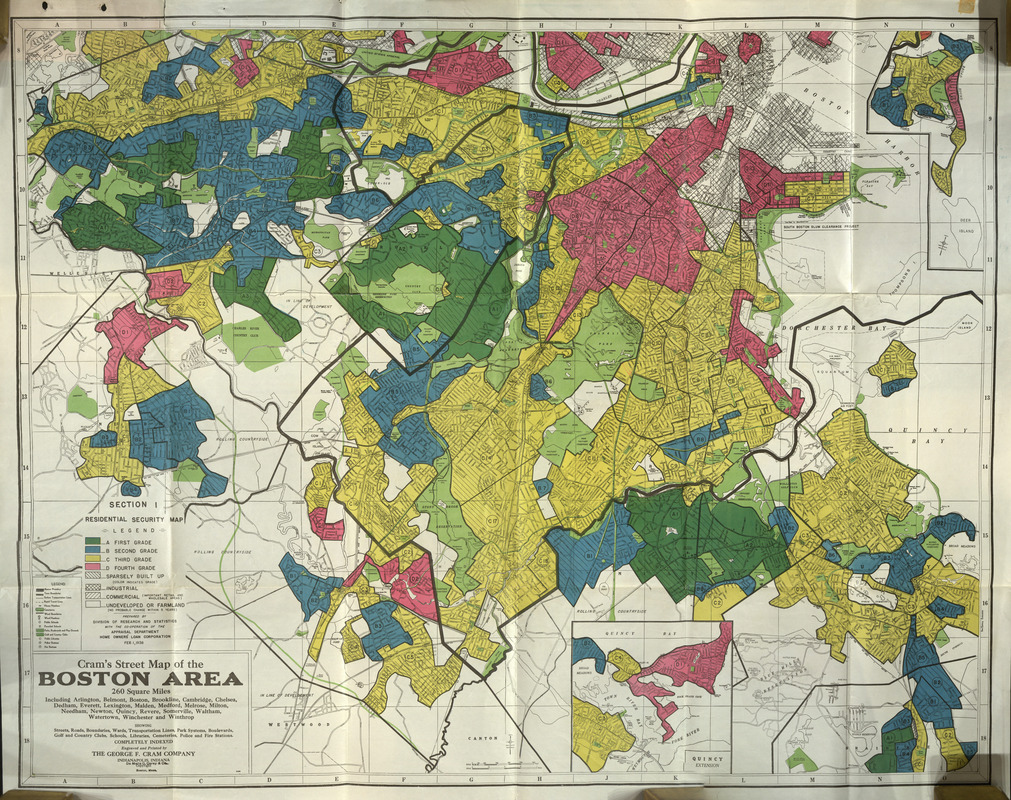
Fairness in Machine Learning (ML) has mostly focused on interrogating the fairness of a particular decision point with assumptions made that the people represented in the data have been fairly treated throughout history. However, fairness cannot be ultimately achieved if such assumptions are not valid. This is the case for mortgage lending discrimination in the US, which should be critically understood as the result of historically accumulated injustices that were enacted through public policies and private practices including redlining, racial covenants, exclusionary zoning, and predatory inclusion, among others.
With the erroneous assumptions of historical fairness in ML, Black borrowers with low income and low wealth are considered as a given condition in a lending algorithm, thus rejecting loans to them would be considered a “fair” decision even though Black borrowers were historically excluded from home-ownership and wealth creation.
To emphasize such issues, we introduce case studies using contemporary mortgage lending data as well as historical census data in the US. First, we show that historical housing discrimination has differentiated each racial group’s baseline wealth which is a critical input for algorithmically determining mortgage loans. The second case study estimates the cost of housing reparations in the algorithmic lending context to redress historical harms because of such discriminatory housing policies.
Through these case studies, we envision what reparative algorithms would look like in the context of housing discrimination in the US. This work connects to emerging scholarship on how algorithmic systems can contribute to redressing past harms through engaging with reparations policies and programs.
RESEARCH AREAS
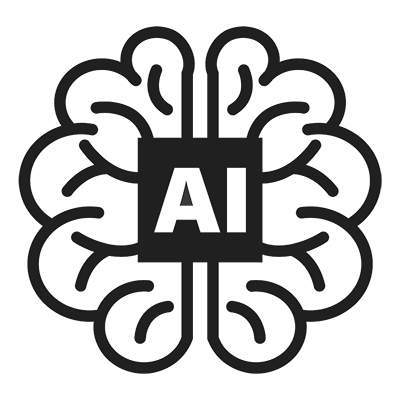
Artificial Intelligence
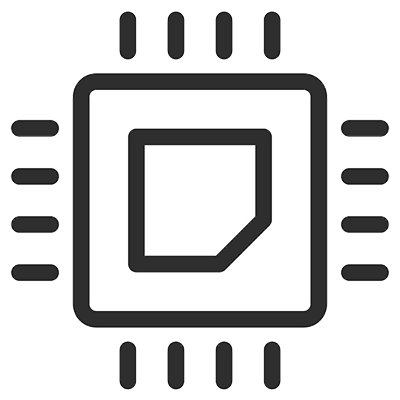
Computation
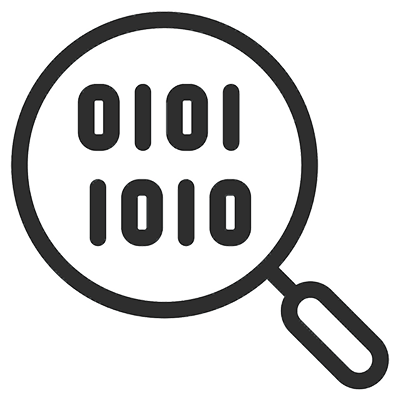
Data Science
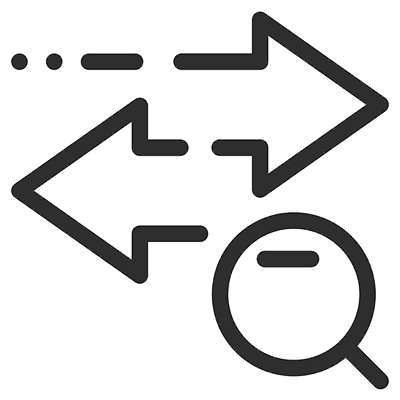
Info & Decision
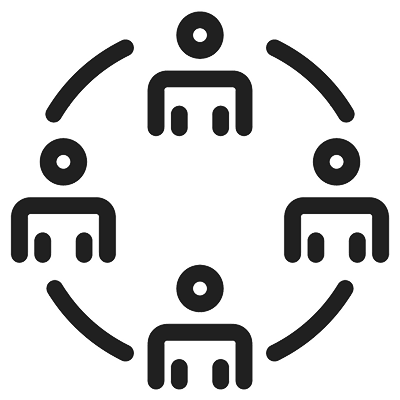
Institutional Behavior
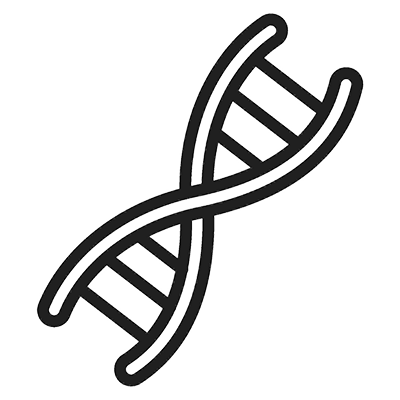
Life Sciences
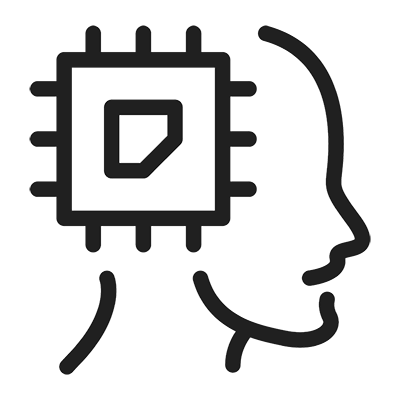
Machine Learning
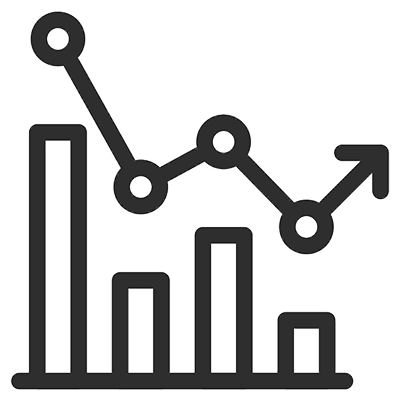
Statistics
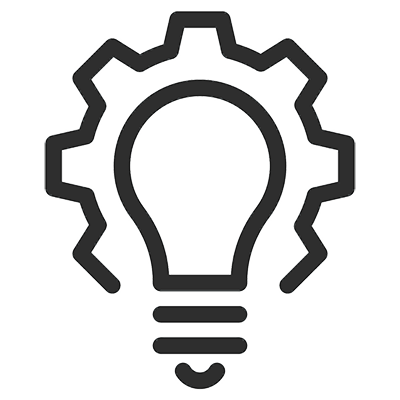
Systems Innovation
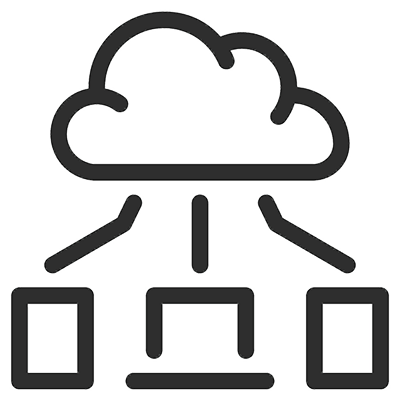
Systems & Networking
IMPACT AREAS
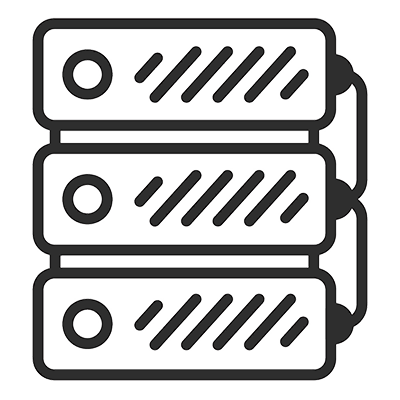
Big Data
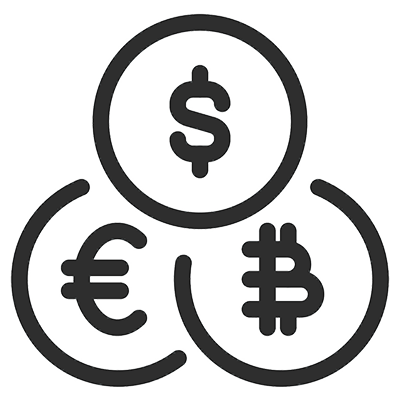
Finance
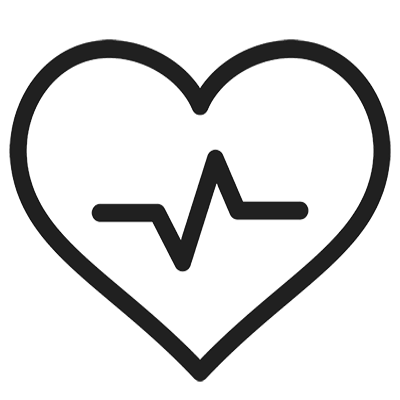
Healthcare / Medicine
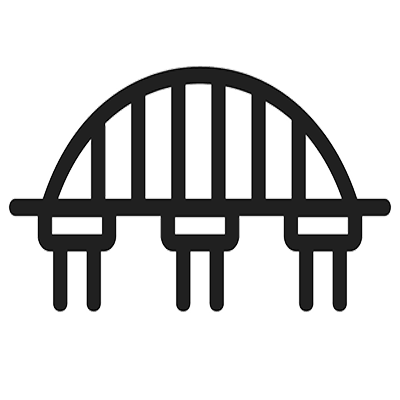
Infrastructure
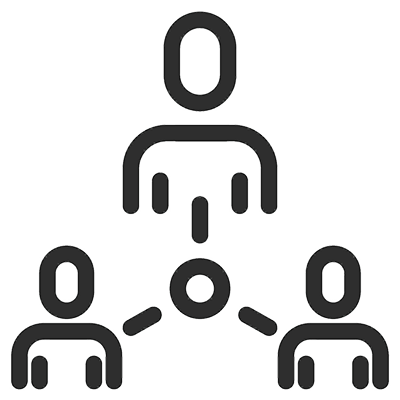
Social Networks
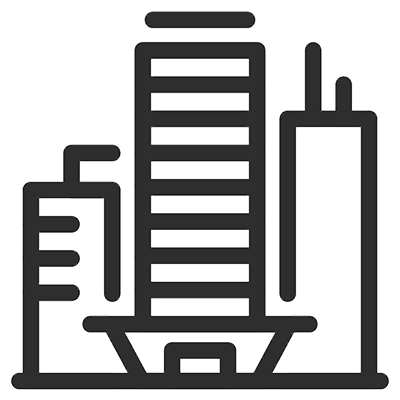