Making data-informed Covid-19 testing plans
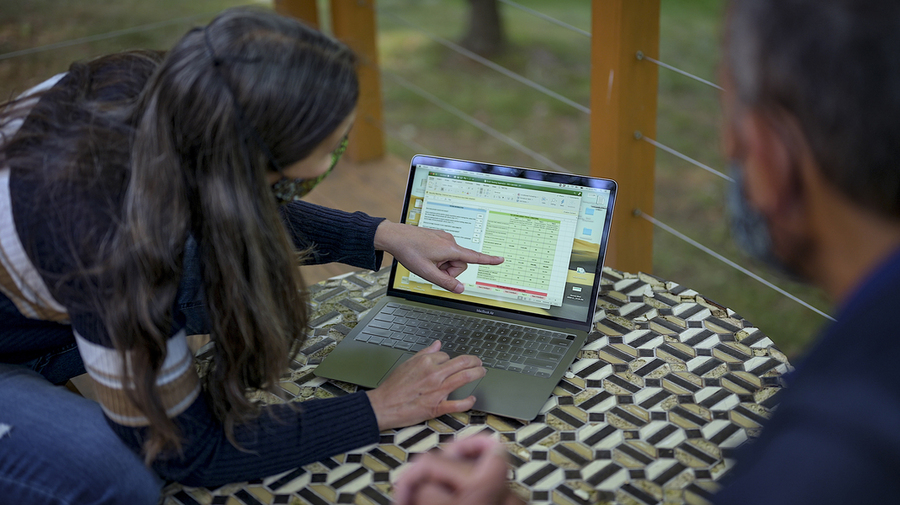
Warehouses, manufacturing floors, offices, schools — organizations of all kinds have had to change their operations to adapt to life in a pandemic. By now, there is confidence in some ways to help mitigate Covid-19 spread: contact tracing, distancing and quarantining, ventilation, mask wearing. And there is one scientific tool that can play a critical role: testing.
Implementing testing within an organization raises a number of questions. Who should be tested? How often? How do other mitigation efforts impact testing need? How much will it all cost? A new web-based Covid-19 testing impact calculator at WhenToTest.org has been developed by MIT researchers with the Institute for Data, Systems, and Society (IDSS), in collaboration with the Consortia for Improving Medicine with Innovation and Technology (CIMIT), to help organizations around the world answer these questions.
“The calculator allows you to do a cost analysis of different trade-offs and enables rational decisions for deploying testing within an organization,” explains Anette “Peko” Hosoi, a professor of mechanical engineering and IDSS affiliate who co-developed the tool. “How much does wearing masks save me? How much does contact tracing save me? How much testing do I have to do if I can’t social distance?”
The web calculator accepts four basic inputs: size of the organization, the percentage of those people who reliably wear masks, whether or not contact tracing is being employed, and the maximum number of people who interact closely without masks. There are also fields for cost considerations, since many organizations will pay to have testing conducted while also paying employees while they are being tested. With this information, the model provides two key estimates: how many people to test daily, and the weekly cost of that testing.
By adjusting the input to these questions, organizational decision-makers can explore these trade-offs, such as increasing mask use and decreasing group size to meet tighter testing budgets. The tool also estimates how much testing should be conducted in situations where masks or distance aren’t possible.
“The powerful thing about this tool is that we are not telling people what to do,” says Hosoi. “We are giving them information that empowers them to make rational, financial decisions that are tailored to their organization.”